2023.11.17 Friday
Accelerating the Phase Identification of Multiphase Mixtures with Deep Learning
Researchers develop a deep learning model that can detect a previously unknown quasicrystalline phase present in multiphase crystalline samples
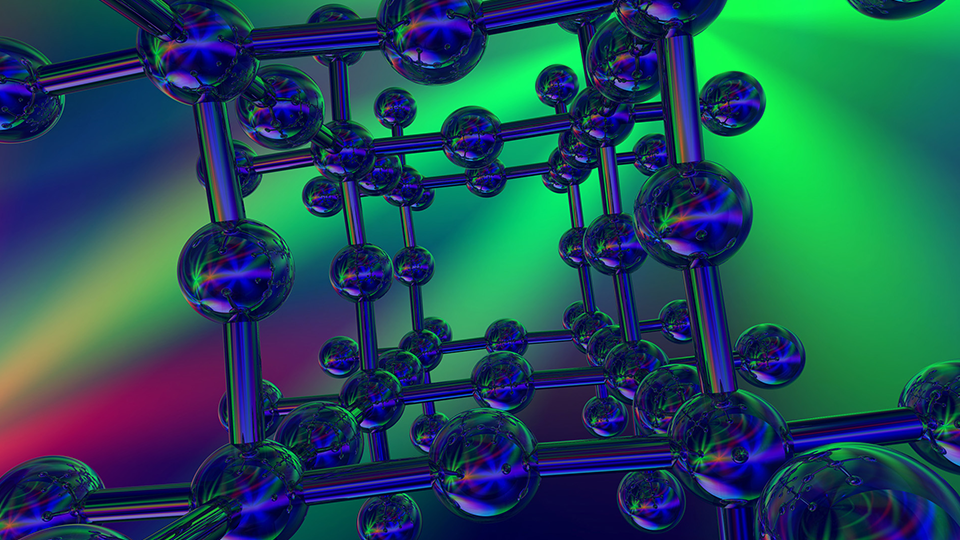
Crystalline materials are made up of atoms, ions, or molecules arranged in an ordered, three-dimensional structure. They are widely used for the development of semiconductors, pharmaceuticals, photovoltaics, and catalysts. The type of structures that fall into the category of crystalline materials continues to expand as scientists design novel materials to address emerging challenges pertaining to energy storage, carbon capture, and advanced electronics.
However, the development of such materials necessitates precise ways of identifying them. Currently, powder X-ray diffraction is widely used for this purpose. It identifies the structure of crystalline materials by examining scattered X-rays from a powdered sample. However, the task of identification becomes quite complex when dealing with multiphase samples containing different types of crystals with distinct structures, orientations, or compositions. In such cases, the accurate identification of the various phases present in the sample relies on the expertise of scientists, making the process time-consuming. To expedite this process, innovative data-driven methods, such as machine learning, have been used for distinguishing individual phases within multiphase samples. While substantial progress has been made in utilizing them for collecting information about known phases, the identification of unknown phases in multiphase samples still remains a challenge.
Now, however, researchers have proposed a new machine learning "binary classifier" model that can identify the presence of icosahedral quasicrystal (i-QC) phases―a kind of long-range ordered solids that have self-similarity in their diffraction patterns―from multiphase powder X-ray diffraction patterns. This study involved collaboration among Tokyo University of Science (TUS), National Defense Academy, National Institute for Materials Science, Tohoku University, and The Institute of Statistical Mathematics. It was led by Junior Associate Professor Tsunetomo Yamada from TUS, Japan, and was published in the Advanced Science journal on 14 November 2023.
"Across the world, researchers have made attempts to predict new substances using artificial intelligence and machine learning. However, identifying whether a desired substance is produced takes up substantial time and effort on the part of human experts. Therefore, we came up with the idea of using deep learning to identify new phases," explains Dr. Yamada.
For developing the said model, the researchers first created a "binary classifier" using 80 types of convolutional neural networks. They next trained the classifier model using synthetic multiphase X-ray diffraction patterns, which were designed as representations of the expected patterns associated with i-QC phases. Following the training phase, the model's performance was assessed using both synthetic patterns and a database of actual patterns.
Quite interestingly, the model achieved a prediction accuracy of over 92%. It also successfully identified an unknown i-QC phase within multiphase Al-Si-Ru alloys when used for screening 440 measured diffraction patterns from unknown materials in six different alloy systems. The presence of the unknown i-QC phase was further confirmed on analyzing the microstructure and composition of the material using transmission electron microscopy.
Notably, the proposed deep learning method has the ability to identify the i-QC phase even when it is not the most prominent component in the mixture. Moreover, this model can be used for the identification of new decagonal and dodecagonal QCs and can be extended to various types of other crystalline materials as well.
"With the proposed model, we were able to detect unknown quasicrystalline phases present in multiphase samples with high accuracy. The accuracy of this deep learning model thus points to the possibility of accelerating the process of phase identification of multiphase samples," concludes Dr. Yamada on an optimistic note. Moreover, Dr. Yamada and his team are confident that this model will lead to a breakthrough in the field of materials science.
In summary, this study is a significant step forward in the identification of entirely new phases in quasicrystals commonly found in materials such as mesoporous silica, minerals, alloys, and liquid crystals.
We surely wish that this model opens up avenues for the discovery of interesting new materials in the future!
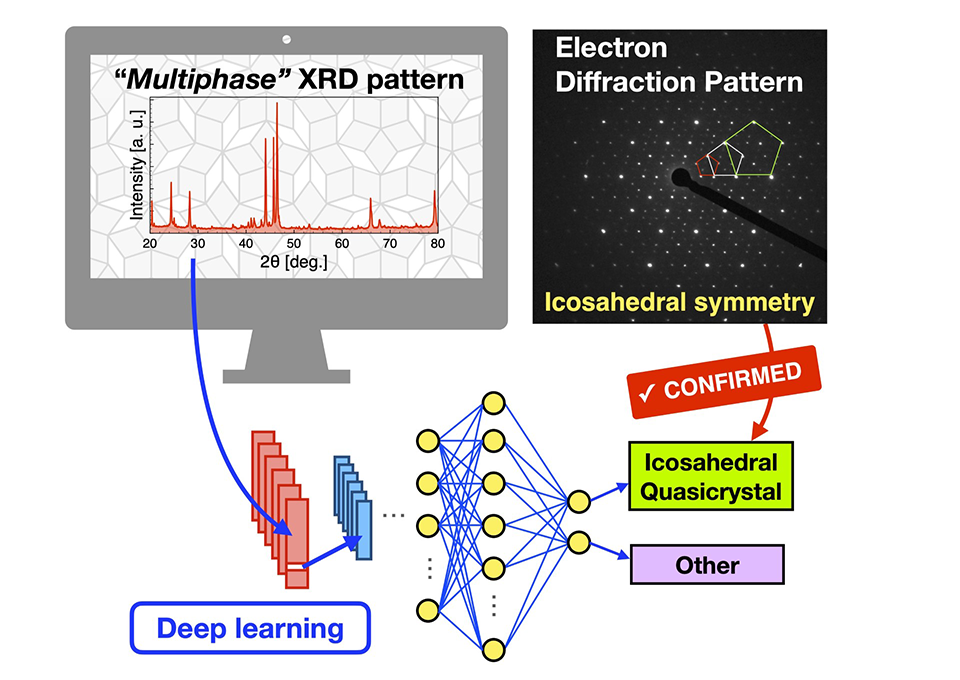
Image title: Researchers develop a new phase identification method for an icosahedral quasicrystal (i-QC) material
Image caption: Researchers propose a machine learning model that identifies a new Al-Si-Ru i-QC phase. With the potential for extended applications for identification of other structures like decagonal and dodecagonal QCs, the proposed model demonstrates immense potential for accelerating the phase identification process of multiphase samples.
Image credit: Tsunetomo Yamada from TUS
License type: CC BY 4.0 DEED
Usage restrictions: Credit must be given to the creator.
Reference
Title of original paper | : | Deep learning enables rapid identification of a new quasicrystal from multiphase powder diffraction patterns |
Journal | : | Advanced Science |
DOI | : | 10.1002/advs.202304546![]() |
Authors: | : | Hirotaka Uryu1, Tsunetomo Yamada1, Koichi Kitahara2,3, Alok Singh4, Yutaka Iwasaki5, Kaoru Kimura5, Kanta Hiroki1, Naoki Miyao6, Asuka Ishikawa7, Ryuji Tamura6, Satoshi Ohhashi8, Chang Liu9, and Ryo Yoshida9,10 |
Affiliations: | : |
1Department of Applied Physics, Tokyo University of Science 2Department of Materials Science and Engineering, National Defense Academy 3Department of Advanced Materials Science, The University of Tokyo 4Electron Microscopy Analysis Station, Structural Materials Microstructure Evaluation Group, National Institute for Materials Science 5Thermal Energy Materials Group, Research Center for Materials Nanoarchitectonics, National Institute for Materials Science 6Department of Materials Science and Technology, Tokyo University of Science 7Research Institute of Science and Technology, Tokyo University of Science, Tokyo 125-8585, Japan 8Institute of Multidisciplinary Research for Advanced Materials, Tohoku University 9The Institute of Statistical Mathematics, Research Organization of Information and Systems 10Department of Statistical Science, The Graduate University for Advanced Studies |
About The Tokyo University of Science
Tokyo University of Science (TUS) is a well-known and respected university, and the largest science-specialized private research university in Japan, with four campuses in central Tokyo and its suburbs and in Hokkaido. Established in 1881, the university has continually contributed to Japan's development in science through inculcating the love for science in researchers, technicians, and educators.
With a mission of "Creating science and technology for the harmonious development of nature, human beings, and society," TUS has undertaken a wide range of research from basic to applied science. TUS has embraced a multidisciplinary approach to research and undertaken intensive study in some of today's most vital fields. TUS is a meritocracy where the best in science is recognized and nurtured. It is the only private university in Japan that has produced a Nobel Prize winner and the only private university in Asia to produce Nobel Prize winners within the natural sciences field.
Further Information
Junior Associate Professor Tsunetomo Yamada
Department of Applied Physics
Tokyo University of Science
Email: tsunetomo.yamada(at sign)rs.tus.ac.jp
Media contact
Hiroshi Matsuda
Public Relations Division
Tokyo University of Science
Email: mediaoffice(at sign)admin.tus.ac.jp
Website
■
Tokyo University of Science(About TUS)
About Junior Associate Professor Tsunetomo Yamada
from Tokyo University of Science
Funding information
This work was supported in part by an MEXT KAKENHI Grant-in-Aid for Scientific Research on Innovative Areas (Grant Numbers 19H05818 and 19H05820), JST CREST Grant (Grant Number JPMJCR22O3), Grant-in-Aid for Scientific Research (A) (Grant Number 19H01132) from the Japan Society for the Promotion of Science (JSPS) and JST CREST Grant (Grant Number JPMJCR19I3).